ADAPT sits at the intersection of systems immunology, synthetic biology, and machine learning. This unique confluence of fields allows the team to attack longstanding problems with fresh eyes and innovative approaches. Harnessing unparalleled experimental scale and data-driven methods, ADAPT aims to lead and advance each of these fields by relying on the diverse skillsets of core members. Potential interventions for ADAPT’s unique approach include the identification and characterization of antibody repertoires, optimization of candidate monoclonal antibodies leads, antigen selection and optimization for vaccine candidates, and enzyme engineering for process improvements. Necessary for ADAPT’s achievement in these areas is to become leaders in the following core technologies:
- Development of AI/ML models: Deep learning algorithms rely on large amounts of data. ADAPT is uniquely suited to generate such data with automation pipelines and established next-generation sequencing/proteomics methods of characterization. ADAPT aims to develop AI/ML models both de novo and from published models with further refinement using our own data sets. Our expertise in experimental design and automation will be instrumental in generating the relevant data needed to build predictive models and answer lingering questions in applied immunology.
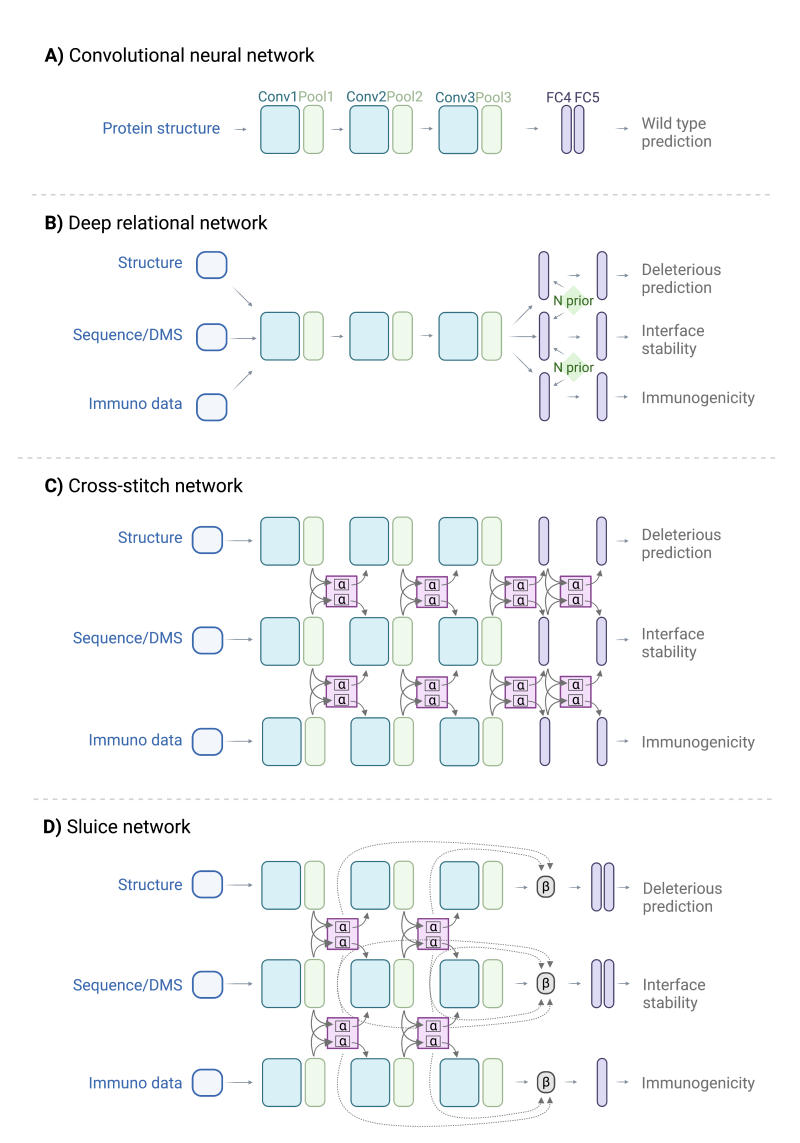
- Computational protein design: With the advent of Alphafold2, the computational protein design field holds great promise. With a high degree of accuracy, we can now predict the structures of previously unsolved proteins. Using Alphafold2 in conjunction with existing software and our own in-house computational tools, we can begin to elucidate mechanisms for binding and epitope prediction, evaluate mutational effects using predicted structures, and screen for fusion proteins and antigen/antibody optimization—all of which will generate hypotheses for further experimental testing.
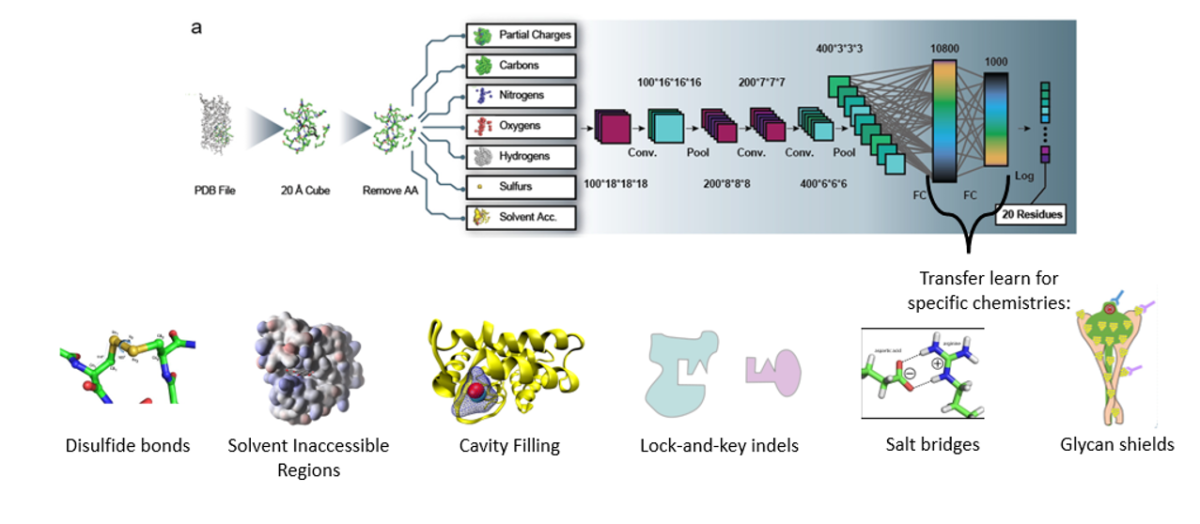
- High-throughput screening and selection: Directed evolution techniques can identify functional variants in massive genetic libraries. We adapt, create, and refine these methods to find the best candidate protein for wide-ranging therapeutic or diagnostic applications. Further, we can use data generated at every round of selection for AI/ML to learn and predict broad evolutionary trends and ultimately engineer proteins.
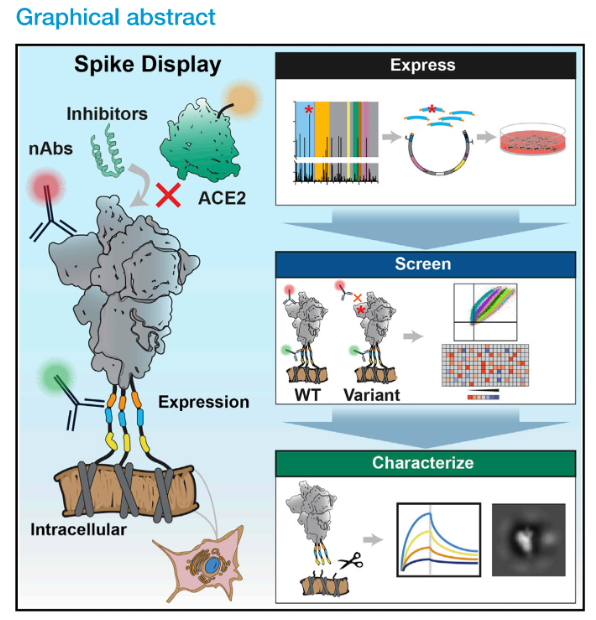
- Turnkey automation pipelines: To undertake many ongoing (and potentially diverse) projects, ADAPT must develop pipelines that are robust and simple enough for a technician to run. Laboratory infrastructure for smooth operation is needed, including sample tracking, lab protocol repository, recordkeeping through electronic lab notebooks, and fail-safes to easily troubleshoot issues. Not only will this ease the burden on senior lab members, but realization of automated pipelines is also necessary to perform experiments at the scale we intend.
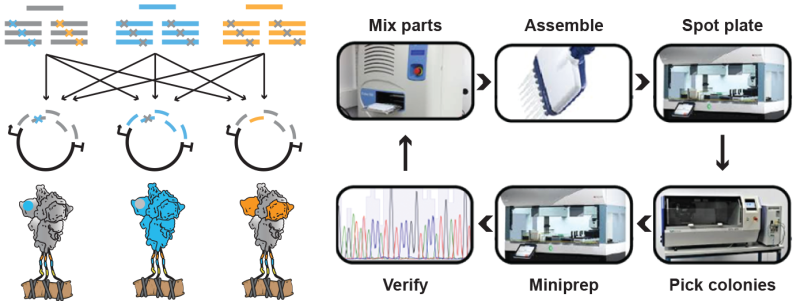